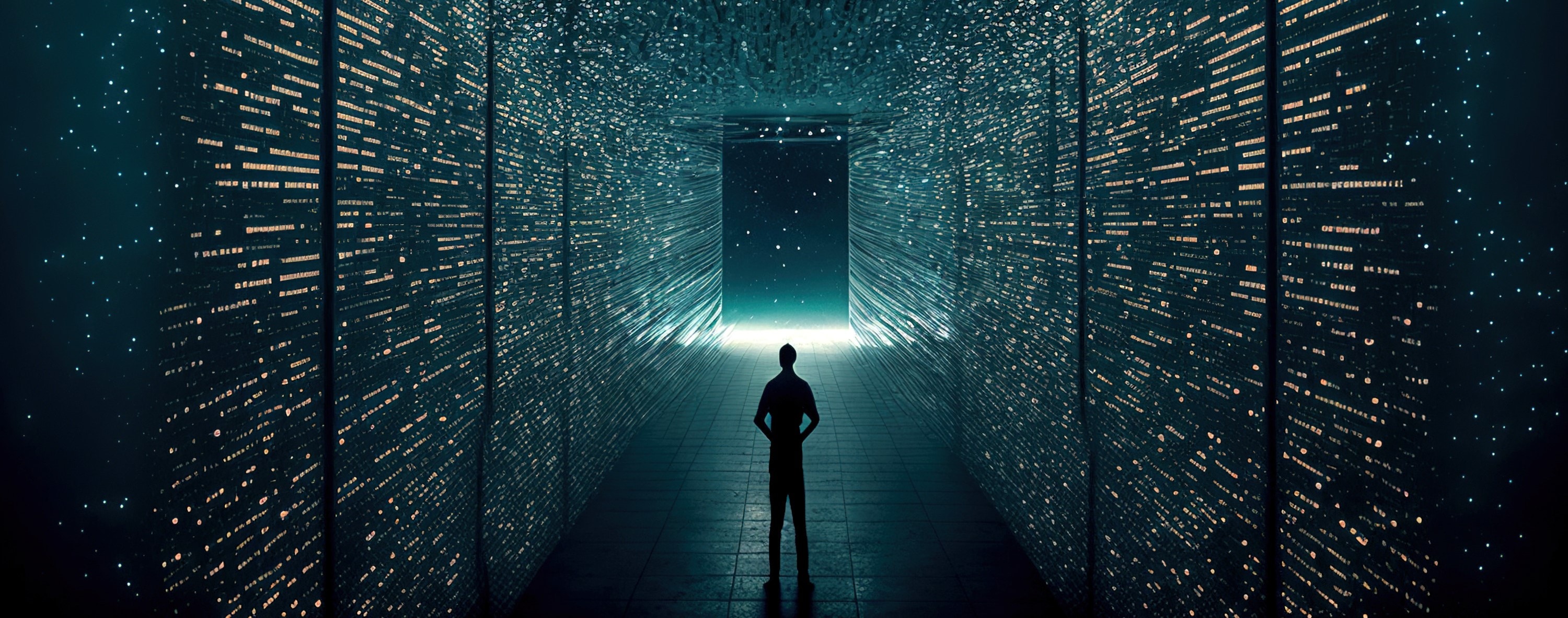
Trend Insight
Understanding Artificial Intelligence
This article explains how AI could change every market, from typical applications to fringe applications that could be impactful to society.
Introduction
Artificial Intelligence, or AI, is everywhere around us in the world. Most of us are familiar with AI in home devices or computers, like Alexa and Siri. And of course, as we join social media, we are constantly given content that is curated to us: we are shown products that we’ve clicked on or have seen in the past. In these applications, AI is a way for machines to learn by themselves and generate responses to provide us with data or services that we want.
Some of the primary use cases for AI are in the marketing realm. If I purchase a certain product on Amazon, Amazon learns my purchase history and may suggest future products. For example, you might see that other customers bought an item because you bought similar products. These ‘recommendation engines’ are really one of the primary use cases for AI.
One of the changes that we see here, though, is that AI is moving into remote and harsh environments, even some applications that require extreme data privacy. Examples include oil and gas exploration, mining and natural resource extraction, farming, food production, water access and sewage control.
How AI Works
AI is based on what we call neural networks. When we feed data to a neural network, we call this machine learning or training. The more data used for training, the smarter the network can be.
Once we train a model, we then deploy that to actual use cases. This is what we call inferencing: I have a model trained, I bring new data to it, and it infers, or expects, a certain outcome based on the data used to train it. And that cycle is repetitive: as the AI engine infers from new data, it is improving its data set. So the more we use features like this, the better – the more things I ask Alexa or use a search engine, the smarter it gets.
We get more precise models, but there are also challenges that come along this. For example, how am I going to store this massive amount of data? How am I going to transfer this data from edge data centers to traditional cloud data centers? If we go back 10 or 15 years, a lot of the data that was created in the world was human-generated data from input, from our keyboards or recordings, film, things like that. And now we are moving into this era of machine-generated data. There’s an absolute data explosion, and that’s certainly influencing how we’re building system architectures and handling this now.
Hardware Advancements Power AI
AI has really taken off in the past ten years because of the evolution of compute hardware that specifically supports it. Nvidia and other companies produce chips that specifically support AI applications, such as graphics processing units (GPUs) or different application-specific integrated circuits (ASICs).
Aside from the drive to develop better GPUs and ASICs for AI, one of the key things is the migration of compute, storage and so-called AI accelerators towards the source of the data. Typically, we have these large data centers; we pin that network and that data is moving to and from those data centers and within those data centers. Now, we are starting to see those compute resources and storage resources moved closer to the edge, to where people and machines are generating the data. This is really the convergence of what we call edge computing with Internet of Things (IoT) endpoints or devices.
AI Challenges: Power and Scalability
There are two key challenges in deploying AI technology: power and scalability. Power is a finite resource; when we look at the footprint of a cloud data center, there’s only so much power that can come into that building and be consumed. So right now, these data centers are not as sustainable as we like them to be. There’s a trend toward sustainable data centers and using renewable energy at these data centers, but we look at AI accelerators, whether they are GPUs or ASICs, they tend to be very power-hungry. This means that on the chip side, designers need to make sure these GPUs and ASICs are power-efficient.
The other side is scalability. We need end sites, edge data centers, and cloud data centers – how do we scale these all of these systems? So-called hyperscale companies like Microsoft, Amazon, Facebook and Google are doing it, but what about the rest of the infrastructure in the world? Just scaling these data centers and systems is an extreme challenge.
Future AI Applications
We have covered the use of AI in personal assistants and e-commerce, but where will AI have a large impact in the future? In reality, every single field is going to be impacted by AI, from manufacturing to transportation to pharmaceuticals.
In the automotive realm, and with driverless cars on the near horizon, we see data center AI technology moving into cars. When we look at what’s in a car today, there’s a variety of sensors and cameras. When you think about how much data a driverless car is collecting, it’s massive. Recently, Nvidia issued some figures out talking about 400 petabytes of raw data with 100 vehicles a year. When we look at the safety and how we test these autonomous vehicles, we want to put them through every possible driving scenario – speeding up around a blind corner, encountering a pedestrian, and so on, to see how the vehicle reacts. The more situations you program into the AI system, the better we can train and tune these models so they’re safe and reliable solutions for transportation, but the data-handling needs are enormous.
Another application is finance – consulting an AI-based app about where we should put our money for retirement and how allocations should be readjusted. The fintech sector is absolutely booming and trying to make these real-time decisions.
In the pharmaceutical industry, developing new drugs is all about iteration – you try something and then test it and try again, sometimes dozens or hundreds of times. AI helps developers learn from previous experiments and apply that knowledge to new ones, ultimately speeding development time.
AI processors get more sophisticated all the time, and AI techniques are continually refined. It may be a while before we have domestic servants like Rosie on the Jetsons, but there’s no doubt that we’re going to encounter AI in many additional aspects of future life.